Experiment 14 - Twin_in_Fog: Data Twin-based and Fog computing driven proactive control and maintenance in Laser-based manufacturing
Experiment description
There is a huge market potential in using advanced data analytics for proactive in-process control and proactive maintenance in laser-based manufacturing, due to the need for learning from data, since the processes are quite complex and vendors are selling machines as "black boxes".
In this experiment it is proposed a novel system for continuous learning from past data, the dynamic models of the machine behaviour, which is based on Fog Computing and requires HPC infrastructure. This experiment will be tested on a challenging 3D laser cutting use case.
Technical description
The use of the 3D laser machine by EMDIP for their high quality cutting services is quite difficult due to its complex system with a number of set points which determine the quality of the output. It is difficult to find an optimal combination of parameters to yield the best quality with the lowest resource utilisation, as well as parameter settings depending on the type and quality of the material in use. The maintenance cost is quite expensive.
With the envisioned process, it is aimed to create a digital twin of the laser cutting machine in the cloud for two reasons:
- to constantly learn models of the machine behaviour in the context the of processes' changes
- to use this models to predict failures and optimize process parameters using reinforcement learning
Based on the digital twin models, it is expected to predict machine failures and issue preventive maintenance warnings, in addition provide set points for the machine to yield best quality and minimal resource utilisation.
Economic Impact
The aim is to achieve 10% market share in support of manufacturing SMEs for laser-based cutting, focusing on Central Europe in the next 2-5 years. The expected savings for EMDIP (end-user) are:
- reduce maintenance cost (30% of annual costs)
- increase OEE (5%) and,
- scrap reduction rate (3%)
Experiment Outcome
This experiment developed a proactive in-process quality control system that can detect anomalies in the operation as soon as they appear (and even before a problem arises). This requires 1) a periodical learning of the new models of usual behaviour and 2) an efficient checking of the model drift by detecting the trends in the new models of the usual behaviour. This enquires an intensive computation which is enabled by the cloud.
The developed solution is a digital twin of the laser cutting machine, learned from past data in the cloud and applied on the edge as a model for classifying new input data - based on D2Twin service which has been developed in the first wave of CloudiFacturing and successfully integrated with the CloudBroker platform.
One of the biggest advantages is that the created Digital Twin, will be automatically updated according to the changes in the data.
Experiment Partners
Competence Centers
Digital Innovation Hub
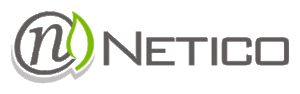
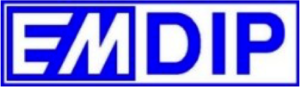
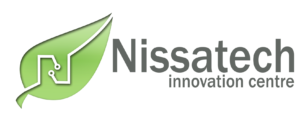


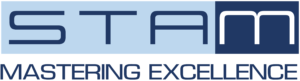