Experiment Description
Chemicals in agriculture can do harm to people's health and the environment. This experiment aims to minimize agrochemicals usage in the industry by optimizing the production process of microcapsules. Microencapsulation is a process in which tiny particles or droplets get surrounded by a coating.
This coating gives many useful properties to those capsules, such as protection, controlled release, specific targeting, improved handling and organoleptic properties which is the reason they are used across a range of industries (e.g. agriculture, food, beverage, pharma and biopharma.).
The main challenge is how to tune each of the input parameters to get the desired properties. End-user Makabi Agritech (MA), a Croatian Bio-Tech Company, currently uses an encapsulator for their daily production needs, facing a costly and time-consuming trial and error process to obtain capsules of desired size and shape. Such an approach also results in the waste of chemicals, which may also represent a negative impact on the environment.
By using high-fidelity Computational Fluid Dynamics (CFD) simulations combined with a neural network trained with Machine Learning (ML) algorithms the prilling process shall be optimized in this experiment. The final goal is to offer a user-friendly Software-as-a-Service (SaaS) tool that will be used by the industry to interact with the digital twin on the cloud.
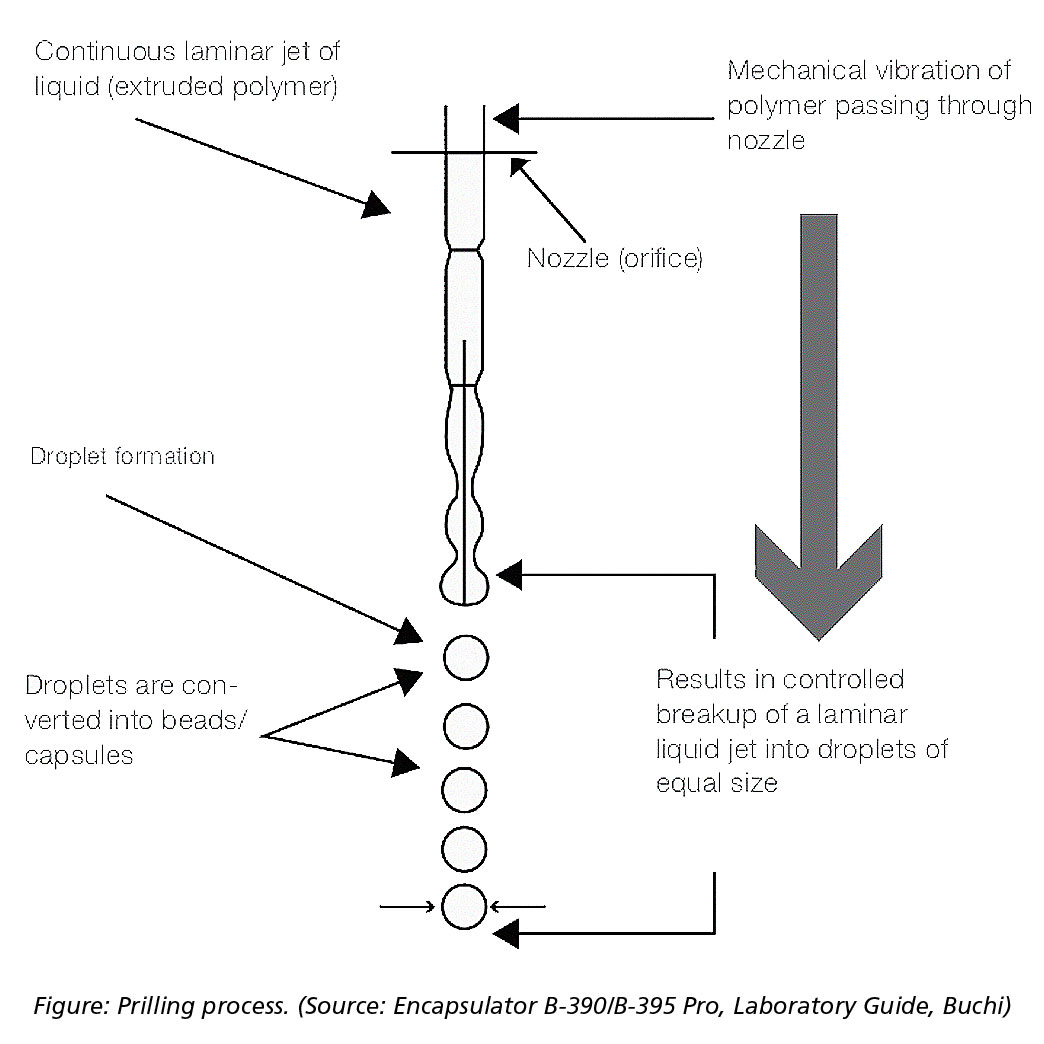
Technical Impact
The digital twin of the prilling process will yield the desired input parameters for the encapsulator based on the desired size of the capsule as specified by the user. The idea is to build an initial neural network based on a limited number of CFD simulations (hundreds instead of several thousand as required by the dimensionality of the input parameters). Such an accurate neural network will provide initial guesses for the queries from the user which will be used to localize the CFD optimization problem.
After the CFD optimization problem is solved on the Cloud, the resulting data will be used in two ways:
- i) The desired input parameters will be provided to the user, and
- ii) The data will be used to enhance the accuracy of the neural network.
Such a physics-based deep learning ML approach will allow Makabi Agritech to provide a service that will become more efficient the more it is being used.
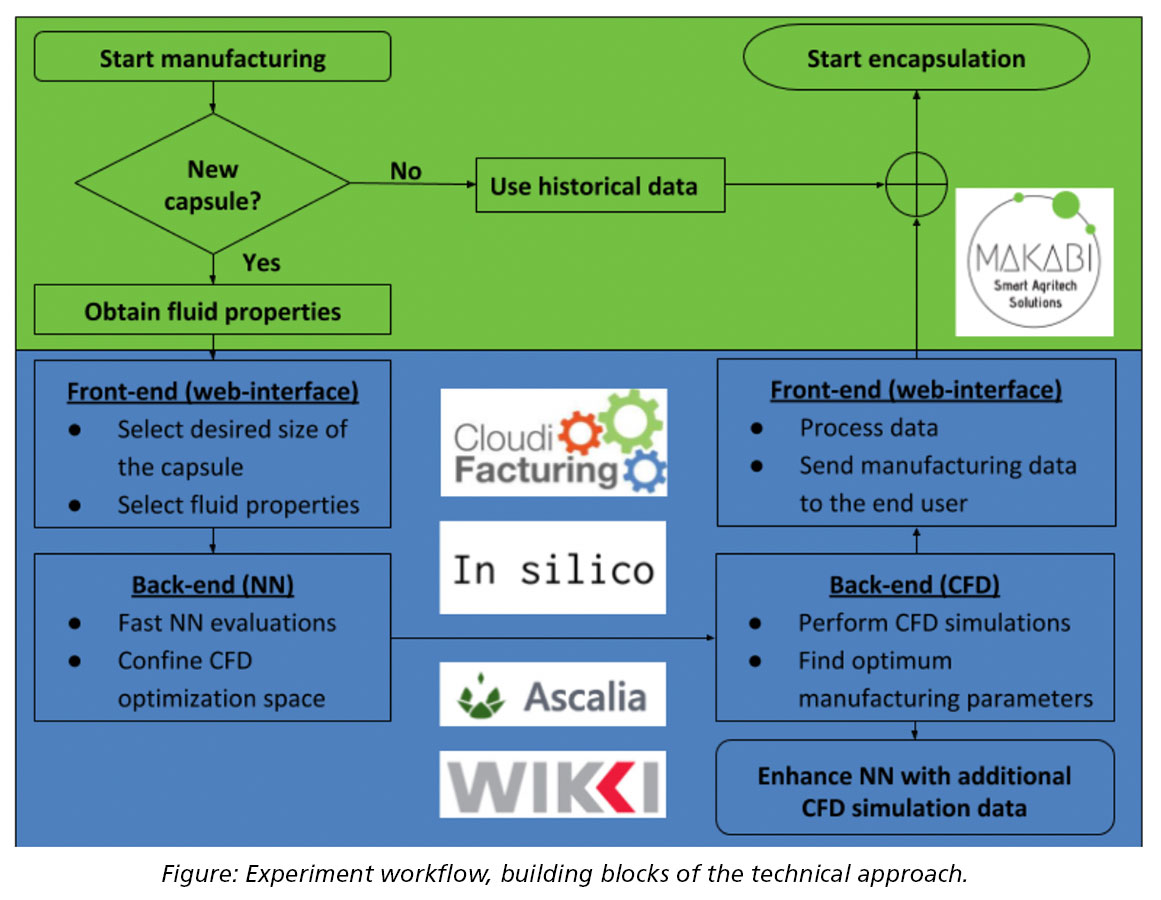
Economic Impact
The final goal of the proposed framework is to allow the end-user to obtain reliable input parameters for the encapsulator to obtain the desired size of the capsules in the shortest amount of time possible. This will directly result in time reduction for the end-user in terms of engineering hours, expecting to save up to two weeks of engineering time per month which will indirectly yield significant cost savings. The developed framework will be suitable not only for the agricultural industry but also for unrelated industries that rely on the encapsulation process and often use similar manufacturing machines.
For Makabi Agritech this solution will provide two important advantages:
1) Faster scale-up through their network of the existing clients,
2) Switching to a recurring revenue business model where this solution could be offered as a subscription part of the manufacturer’s sales.
Offering such an enhanced SaaS Solution to their existing, but different sets of clients, MA will be able to pass on cost advantages to them. After initial validation on regional market, the broader European market will be targeted.
Experiment Partners
Competence Centers
Digital Innovation Hub
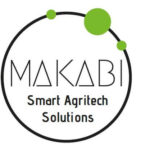
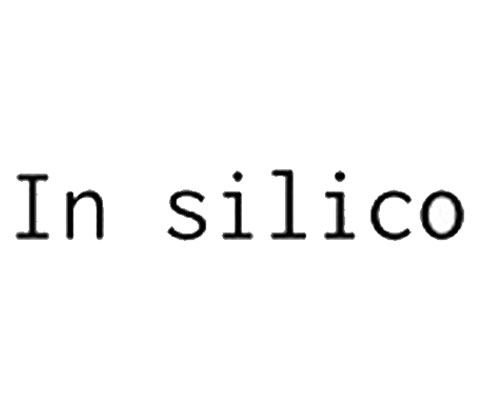
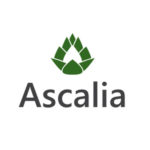
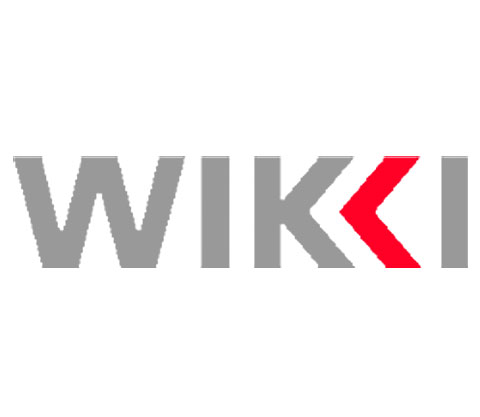
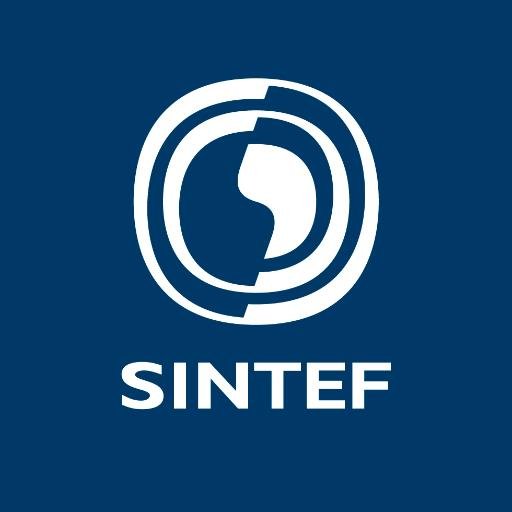
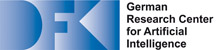
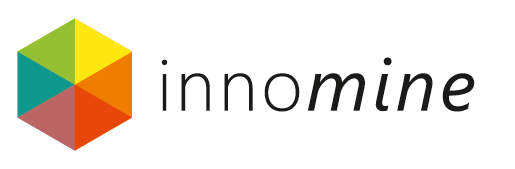