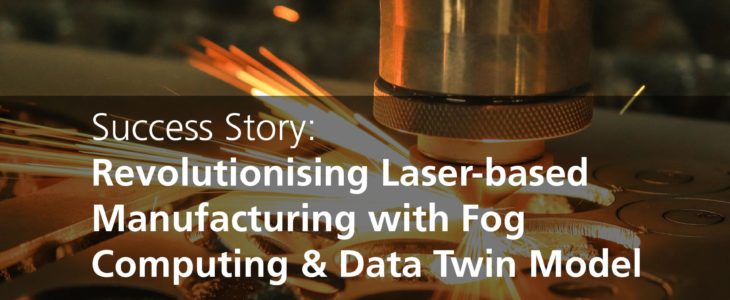
TwinInFog: Revolutionizing Laser-based Manufacturing through data twin-based and fog computing driven proactive control and maintenance
Success Story
MOTIVATION
Laser cutting is one of the leading applications in the field of laser-based manufacturing, also very well adopted by manufacturing SMEs. The laser technology ensures high quality in the manufacturing process as the laser-cutting machines are very precise. Unfortunately, they’re quite expensive, too. Moreover, this sector is suffering from very high maintenance costs, since every vendor is offering exclusive maintenance service after warranty period. This paradoxically entails that the total maintenance costs after 7-8 years of operation can be equivalent to a third of the price of the new machine.
EMDIP, the end user company in CloudiFacturing’s Experiment 14, is a manufacturing SME that uses laser-cutting technology to offer a wide range of high quality metal cutting services. Due to the need for expert modelling and analysis which is expensive and time consuming, EMDIP has been hesitant in implementing formal quality models and process monitoring tools. Although the company uses the most advanced machines, they have experienced two problems which cannot be easily resolved using traditional methods:
- how to ensure an efficient and less cost intensive maintenance process of a machine that usually has been used very intensively and heavily in the specific conditions (wearing process can be substantial, including de-calibration).
- how to ensure high quality of the production process that is influenced by a plenty of different parameters (including contextual parameters such as environmental temperature) when the knowledge on how these parameters influence the process doesn’t appear to exist (in the user manuals) in an explicit form.
These problems relate to the efficient usage of resources that should be controlled in the real-time in order to ensure the desired quality. But real-time control and integration of information from different sensors is a difficult task, since laser-based machines operate with peak performance in order to satisfy high quality requirements for the laser-based processes (e.g. 3D/2D cutting, welding).
THE CHALLENGE
A laser cutting system is primarily defined by the type of laser source used to generate the laser beam. The machines, EMDIP currently uses are accompanied with the software that uses the data produced by sensors and provide different views on the operation processes. However, this data is used only for checking the performance of machines, but not the quality of the products.
The main challenge in this experiment was that the resolution of above mentioned problems requires deep knowledge about the particular/individual process and the used machines/equipment, e.g. how a particular machine behaves when the speed of rotation is increasing (e.g. how is the temperature changed) and how the temperature in that particular room is influencing the cutting process executed in that particular machine. Such knowledge mostly cannot be found in the user manuals and even vendors cannot say much about the causes of a particular wearing process without doing some additional observations on the particular machine.
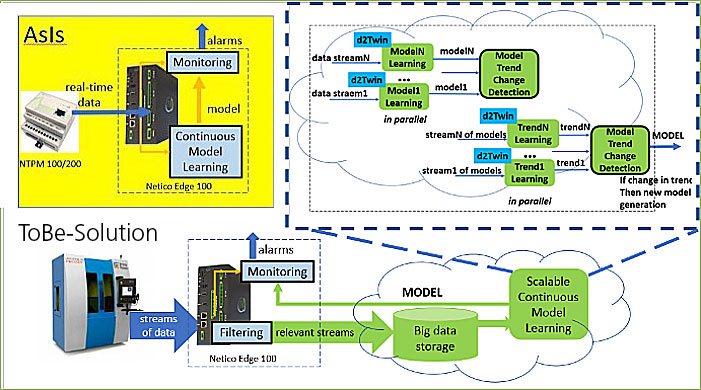
GOALS OF THE EXPERIMENT
The solution: TwinInFog, which is Experiment No. 14 in the CloudiFacturing Project, is a complex Fog Computing system that senses from the edge, sends the data to the cloud where a very complex learning is performed and returns models for the complex predictions on the edge. The system is used to reach the following goals:
- Exploitation of the value of data: getting a deep understanding of the behaviour of a concrete manufacturing asset (machine), based on the intelligent analytics (beyond deep learning and data analytics) on the past data.
- Data-driven maintenance: exploit the power of data for a more efficient maintenance process that is independent of the vendor, data-driven and more efficient.
- Make a digital replica (individualized monitoring and control): create Digital Twins, as digital replicas of physical assets (machine). Such a model provides new possibilities for monitoring and controlling quality of the products as well as the wear process of machine, but in a very individual way (instead of the vendor prescribed one-size-fits-all model, so called “model by design”), opening new possibilities for optimization.
TECHNICAL IMPACT
The main outcome of the experiment is a proactive in-process quality control system that is able to detect anomalies in the operation as soon as they appear or even before, but without any a-priori knowledge about the complex operation. Indeed, such knowledge exists only for univariate analysis (one parameter). Multivariate analytics learning process is applied. The assumption was that the problems in the operation are reflected on the values of the parameters of the process through showing some unusual behaviour. However, since the concept of the “unusual” can be changed according to the trends in the input data, the system enables:
- periodical learning of the new models of usual behaviour;
- efficient checking of the model drift by detecting the trends in the new models of the usual behaviour.
Such a generalization of knowledge requires the application of AI techniques which will transform the past data in the operational memories of the machine (and the whole production system), i.e. the models which reflect the past behaviour of the machine and are able to reason about the new cases (incl. unknown). It seems like a virtual replica of the behaviour of that particular machine, which is by definition a digital twin. The basis for the processing (model learning) is D2Twin which has been developed in the first wave of CloudiFacturing and successfully integrated with the CloudBroker platform.
ECONOMIC IMPACT
The main benefit that this experiment aims to bring is that manufacturers will be able to exploit the power of data towards an improved maintenance process:
-
-
- Independent from the laser cutting machine vendor to decrease maintenance costs;
- More efficient, to be measured as an increase of OEE (overall equipment efficiency).
-
Thanks to this improved service, new customers are expected to be attracted at EMDIP through an improved quality of maintenance for a lower price.
The service resulting from this experiment is intended to be used by other SMEs who are in the same situation as EMDIP. Most of them consider the current maintenance costs, that are paid to the machine vendor, as extremely high and would be ready to immediately test another solution that will make them independent from the machine vendors. In this case the actual pricing model of the solution is proportional to the cost of savings a customer realizes. Indeed, this offer is based on a new solution for managing maintenance processes in a completely different way, shifting the mindset that the “maintenance is a cost” into “maintenance is an opportunity for the cost cutting and even process improvement”.
The global laser-cutting machine market size is expected to grow by 1.25 billion USD during 2019-2023 with a CAGR of 9.7% over the next five years. Europe is still the largest production market of laser-cutting machine products (26.78%) followed by North America (24.76%). Therefore, many industries who are dealing with laser cutting, such as automotive, aerospace and defence, electrical and electronics, industrial machinery, etc., will definitely benefit from this solution.